19 Teaming with Machines
Cameron W. Piercy
I bet you read the chapter title and thought: What? Machines? I am probably going to work in an office, with people. That’s likely, even during the pandemic, 64% of high school graduates want to work in offices (Maurer, 2021). But, consider this about 25% of all U.S. homes and 1/3 of 18-29 year-olds have a smart speaker in their home (and 54% of owners say ‘please’ to their device, more on that later; Auxier, 2019). As of writing, algorithmic calendar applications are on the rise seamlessly scheduling meetings (on Zoom, Teams, or other mediated platforms of increasing ‘intelligence’ in real time), by assessing both of our digital calendars avoiding conflicts, and it will email us both a link. Software like this and even Google’s proprietary (that means it’s their property and they don’t have to explain how it works) algorithm affects how we do our jobs. Further, whether you work in an office with people, on a factory floor, or whatever context you are likely to team with machines as part of the future of work.
Machines are already being used to teach courses (Abendschein et al., 2021a; C. Edwards et al., 2018), as pets (A. Edwards et al., 2021), for eldercare (Chang & Šabanović, 2015), and for many other purposes. In each of these applications, complex machines or machines capable of abstracting principles to guide function including algorithms, artificial intelligence, machine learning models, robots, and other forms, are increasingly moving from tool to teammate. Don’t believe me? Open and email and start typing—if you are using a modern email client, it’s very likely a machine is analyzing your writing as you write and recommending how you finish the sentence. While this may seem novel, machine collaboration has been steadily increasing for years. As I write this paragraph each misspelling (relatively) seamlessly autocorrects to amend my spelling and grammar.
So, what does all this mean? Why does ‘teaming with machines’ matter? This chapter begins the conversation, while inviting you to imagine the work you are already doing in teams and the work you will be doing in teams that will likely include complex machines.
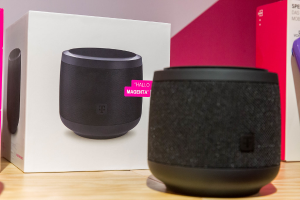
Problematizing Teaming with Machines
Like much of this OER, I want to problematize (that is to frame the potential issues and benefits) the process of teaming with machines for you. Sebo et al. (2020) put it clearly, the “literature provides compelling evidence that people interact differently with robots when they are alone than when they are with other people” (p. 176:4). There are several key mechanisms which guide human behavior when working with complex machines: the Computers as Social Actors (CASA) paradigm and the MAIN (modality, agency, interactivity, and navigability) model. The chapter ends by focusing on evidence of machines as members of teams.
Computers as Social Actors (CASA)
Though the design of a technology (e.g. level of interactivity, cues) affects how we interact with technologies, people generally use human-human interaction scripts to interact with technologies (see, Reeves & Nass, 1996; Lombard & Xu, 2021). In both novel and mundane situations, people form and act on impressions of technology that are based on a longstanding psychological tendency towards anthropomorphizing the physical world (Broadbent, 2017; Fortunati & Edwards, 2021). This tendency is captured well in the computers as social actors or CASA paradigm, which claims we are not evolutionarily adapted to machines and so we apply patterns of human interactions to machines (Reeves & Nass, 1996) even those with extremely limited communication (e.g., industrial robots; Guzman, 2016).
CASA is undergirded with three assumptions (1) humans are social creatures and our brain is adapted for and attuned to social interaction (Banks, 2020); (2) humans are naturally interdependent and cooperative (Brewer, 2004; Tomasell, 2014); and (3) “humans have evolved to develop the propensity for flexibility in using social cues during communication and cooperation with others” (Lombardi & Xu, 2021, p. 41). The CASA paradigm argues human brains have developed to be attuned to social interaction, so humans generally use the same cognitive scripts to interact with machines as they do with humans (Nowak et al, 2015; Reeves & Nass, 1996). This is not to say interaction with machines are thoughtful; indeed, they are often mindless for both human and machine communication partners (Gambino et al., 2020). However, this finding suggests that validated social theory ought to apply well to new sociotechnical HMC relationships (de Graaf & Malle, 2019; Westerman et al., 2020). The ubiquitous CASA findings, that people use overlearned behaviors to interact with machines as they do with humans, invites scholars to translate theory to in situ work with machines. Thus, the CASA paradigm serves as a powerful explanation guiding how humans evaluate machine communication partners.
The MAIN (modality, agency, interactivity, and navigability) Model
Sundar (2008) proposed the MAIN (modality, agency, interactivity, and navigability) model for assessing credibility of information in complex online environment. MAIN argues that cues transmitted via technological affordances prompt heuristic evaluations of information and yield credibility judgments. Specifically, affordances refer to perceived capabilities of a technology. For example, Roomba’s are known for their ability to vacuum. But, you may also know their ability to spread dog poop around a house. Similarly, Amazon’s Echo devices are good at answering simple questions, playing games, and offering shopping suggestions. Echo’s can even be configured to turn on and off lights, open garage doors, monitor for broken glass, or unlock house doors. But, they cannot provide a hug.
Cues from technology can be as simple as consensus by users (e.g., likes on Facebook, upvotes on Reddit) or as complex as conversations with Siri. MAIN focuses on four categories of affordances that prompt evacuation and lead to credibility assessments of a given partner (both humans and machines). Modality are the most structural of affordances—it refers to the text, audio, video, or other sensory form of communication available in a given technology. For example, Zoom offers video, audio, and text-based communication, whereas chat is limited to text, emojis, and gifs.
Agency asks who or what is responsible for the message? Agency focuses on the source of action—be it algorithm, person, robot, or other source. For example, when you read a news article you might ask who or what wrote this. Today, news articles are increasingly (and successfully) being writing by AI (see Guzman & Lewis, 2020).
Interactivity implies both interaction and activity—and focuses on a given technology’s ability to involve or engage the users. The easiest example might be comparing a simple 8-bit video game, like Pacman, with a complex virtual environment available on Oculus or in Meta. At a different level, Dora the Explorer is more interactive than Scooby Doo, because it invites viewers to respond to the media (Sundar, 2008). Like the other MAIN components, interactivity affects how users assess credibility.
The final cue in the MAIN model is navigability, which focuses on “interface features that suggest transportation from one location to another” (Sundar, 2008, p. 88). Though Sundar does not spend significant time on functionality as a category of navigability—I reflect on my own use of technology tools that did or did not work so well to think about this affordance. Consider your frustration using a call system that does not recognize your voice—or, in contrast, finding the perfect piece of information.
Each affordance, in turn, prompts heuristics, or attentional shortcuts which guide evaluations, for the technology and source of information. For example, when a post has many positive reactions (regardless of content) we trust it, which is called the bandwagon heuristic. Evidence suggests that the number of likes or retweets signals the bandwagon heuristic. Lin and Spence (2018) tested the bandwagon heuristic and another heuristic, the identity heuristics of tweets. To test the identity heuristic, they changed the identity of the person tweeting (stranger, student peer, or FDA expert). Their results validate the MAIN model for perceptions credibility of the message—showing the expert was rated higher than a peer which was higher than a stranger.
As you can tell, heuristics are prompted easily (e.g., number of likes/retweets, identity of speaker). The details of the many heuristic processes prompted by affordances is beyond the scope of this chapter. But, Figure 1, reproduced directly from Sundar (2008, p. 91) shows the full MAIN model and how affordances prompt heuristics which influence quality assessments and credibility judgements.
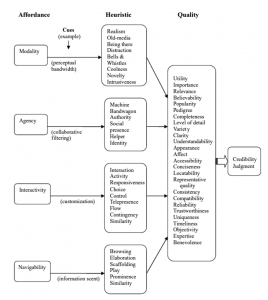
So, why does MAIN matter? Well, every machine has modality, agency, interactivity, and navigability components. When you find yourself liking, disliking, or feeling indifferent toward a machine partner—you are engaging in quality assessments and credibility judgments. These are not the only or most important components of interaction with machines, but they do affect our interaction with machines.
Team Composition and Machines
Machines can serve many roles in HMC networks and may be more or less numerous than human counterparts (Tsarouchi et al., 2016). The composition of the human-machine team also matters. Though research on mixed human-machine teams is only emerging, studies show that the more non-human machines in a group the higher outgrouping by human and more human competition (Fraune et al. 2019; Xu & Lombard, 2017). Further, when interacting with robots in a group humans report more negative emotions and greater anxiety (Fraune et al., 2019). At the same time, evidence suggests that workers are more willing to cede decision-making to robots when the robot is efficient (Gombolay et al., 2015). Further, people prefer to use algorithms when they agree with existing beliefs (Mesbah et al. 2021) and prefer to use search engines over asking peers (Oeldorf-Hirsch et al., 2014). Bots also elicits reduced reciprocity evaluations (Prahl & van Swol, 2021).
In an experiment which prompted users to select between options in collaboration with complex computer agents, users were influenced by the computers. Specifically, Xu and Lombard (2017) found that the more users perceived the computers as ‘close to human’ the more likely they were to agree with the computer. Further, when text of the computer program matched the color of the icon for the users text (e.g., computer and user both display in blue text) the users were more likely to agree with the computer, likely signaling a shared social identification with the machine.
There is a large foundation of group decision support software (GDSS) by Scott Pool and Geraldine DeSanctis among others (1990; 1994). GDSS is discussed elsewhere in the text, but this software was useful in understanding how technology and people interact. MicBot is an excellent and succinct example of how machines can alter human communication processes. Tennent, Shen, and Jung (2019) created MicBot as a bot that moves the microphone between group members to facilitate more egalitarian conversation. Specifically, Tennent et al. (2019) concluded MicBot “increased group engagement but also improved problem solving performance” (p. 133). MicBot did just that—the bot is simple, but (as you have learned if you have read other chapters), this systematic change can lead to large effects.
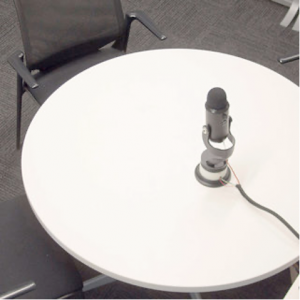
The findings about the role of machines, bots, AI, in teams and groups is still emerging. Sebo et al. (2020) in their comprehensive literature review offer a solid conclusion of the knowledge at the time this chapter was written:
In summary, the literature provides compelling evidence that 1) robots cannot simply be conceptualized as tools or infrastructure, but also are not always viewed similarly to people, 2) people interact differently with robots when they are alone than when they are with other people, 3) that a robot’s behavior impacts how people interact with each other, and 4) that while current findings on group effects are consistent with theory on human-only groups, it might be premature to assume that this is always the case given that most research up to this point relied on anthropomorphic robotic systems. (p. 176: 19).
Machines are more than tools, robots who are members of teams affect team dynamics both with the machine and with fellow humans, and as novel technologies emerge we need to continue to attend to how they affect our team and group interaction. Evidence shows that machines affect our communication with one another and our communication with machines. Key theories like CASA tell us how people treat machines, and explain why we are often polite to machines (Auxier, 2019).
References
Abendschein, B., Edwards, C., & Edwards, A. (2021a). The influence of agent and message type on perceptions of social support in human-machine communication. Communication Research Reports, 38(5), 304-314.
Abendschein, B., Edwards, C., Edwards, A. P., Rijhwani, V., & Stahl, J. (2021b). Human-Robot Teaming Configurations: A Study of Interpersonal Communication Perceptions and Affective Learning in Higher Education. Journal of Communication Pedagogy, 4(1), 12.
Banks, J. (2020). Optimus primed: Media cultivation of robot mental models and social judgments. Frontiers in Robotics and AI, 7, 62.
Brewer, M. B. (2004). Taking the social origins of human nature seriously: Toward a more imperialist social psychology. Personality and Social Psychology Review, 8(2), 107–113. https://doi.org/10.1207/s15327957pspr0802_3
Broadbent, E. (2017). Interactions with robots: The truths we reveal about ourselves. Annual Review of Psychology, 68, 627-652. https://doi.org/10.1146/annurev-psych-010416-043958
Chang, W. L., & Sabanovic, S. (2015, March). Interaction expands function: Social shaping of the therapeutic robot PARO in a nursing home. In 2015 10th ACM/IEEE International Conference on Human-Robot Interaction (HRI) (pp. 343-350). IEEE.
de Graaf, M. M., & Malle, B. F. (2019, March). People’s explanations of robot behavior subtly reveal mental state inferences. In 2019 14th ACM/IEEE International Conference on Human-Robot Interaction (HRI) (pp. 239-248). IEEE. https://doi.org/10.1109/HRI.2019.8673126
DeSanctis, G., & Poole, M. S. (1994). Capturing the complexity in advanced technology use: Adaptive structuration theory. Organization science, 5(2), 121-147.
Edwards, A., Edwards, C., Abendschein, B., Espinosa, J., Scherger, J., & Vander Meer, P. (2020). Using robot animal companions in the academic library to mitigate student stress. Library Hi Tech.
Fortunati, L., & Edwards, A. P. (2021). Moving Ahead With Human-Machine Communication. Human-Machine Communication, 2, 7-28. https://doi.org/10.30658/hmc.2.1
Fraune, M. R., Šabanović, S., & Kanda, T. (2019). Human group presence, group characteristics, and group norms affect human-robot interaction in naturalistic settings. Frontiers in Robotics and AI, 6, 48. https://doi.org/ 10.3389/frobt.2019.00048
Gambino, A., Fox, J., & Ratan, R. A. (2020). Building a stronger CASA: Extending the computers as social actors paradigm. Human-Machine Communication, 1, 71-86. https://doi.org/10.30658/hmc.1.5
Gombolay, M. C., Gutierrez, R. A., Clarke, S. G., Sturla, G. F., & Shah, J. A. (2015). Decision-making authority, team efficiency and human worker satisfaction in mixed human–robot teams. Autonomous Robots, 39(3), 293-312. https://doi.org/10.1007/s10514-015-9457-9
Guzman, A. L. (2016). The messages of mute machines: Human-machine communication with industrial technologies. Communication+1, 5, 1-30. https://doi.org/10.7275/R57P8WBW
Guzman, A. L., & Lewis, S. C. (2020). Artificial intelligence and communication: A Human–Machine Communication research agenda. New Media & Society, 22(1), 70-86. https://doi.org/10.1177/1461444819858691
Lin, X., & Spence, P. R. (2019). Others share this message, so we can trust it? An examination of bandwagon cues on organizational trust in risk. Information Processing & Management, 56(4), 1559-1564.
Lombard, M., & Xu, K. (2021). Social Responses to Media Technologies in the 21st Century: The Media are Social Actors Paradigm. Human-Machine Communication, 2, 29-55. https://doi.org/10.30658/hmc.2.2
Maurer, R. (2021, July 18). The Class of 2021 Wants to Work in the Office. Society of Human Resources Management. https://www.shrm.org/resourcesandtools/hr-topics/talent-acquisition/pages/the-class-of-2021-wants-to-work-in-the-office-hybrid-flexible-salaries.aspx
Mesbah, N., Tauchert, C., Buxmann, P. (2021). Whose advice counts more – man or machine? An experimental investigation of ai-based advice utilization. In Proceedings of the 54th Annual Hawaii International Conference on System Sciences (HICSS). https://hdl.handle.net/10125/71113
Nowak, K. L., Fox, J., & Ranjit, Y. S. (2015). Inferences about avatars: Sexism, appropriateness, anthropomorphism, and the objectification of female virtual representations. Journal of Computer-Mediated Communication, 20, 554-569. https://doi.org/10.1111/jcc4.12130
Oeldorf-Hirsch, A., Hecht, B., Morris, M. R., Teevan, J., & Gergle, D. (2014, February). To search or to ask: The routing of information needs between traditional search engines and social networks. In Proceedings of the 17th ACM conference on Computer supported cooperative work & social computing (pp. 16-27). https://doi.org/10.1145/2531602.2531706
Poole, M. S., & DeSanctis, G. (1990). of Group Decision Support Systems: The Theory of Adaptive Structuration. Organizations and communication technology, 173.
Prahl, A., & Van Swol, L. (2021). Out with the humans, in with the machines?: Investigating the behavioral and psychological effects of replacing human advisors with a machine. Human-Machine Communication, 2, 209-234. https://doi.org/10.30658/hmc.2.11
Reeves, B., & Nass, C. I. (1996). The media equation: How people treat computers, television, and new media like real people and places. Cambridge University Press.
Sebo, S., Stoll, B., Scassellati, B., & Jung, M. F. (2020). Robots in groups and teams: a literature review. Proceedings of the ACM on Human-Computer Interaction, 4(CSCW2), 1-36.
Sundar, S. S. (2008). The MAIN model: A heuristic approach to understanding technology effects on credibility (pp. 73-100). MacArthur Foundation Digital Media and Learning Initiative.
Tennent, H., Shen, S., & Jung, M. (2019, March). Micbot: A peripheral robotic object to shape conversational dynamics and team performance. In 2019 14th ACM/IEEE International Conference on Human-Robot Interaction (HRI) (pp. 133-142). IEEE.
Tomasello, M. (2014). The ultra-social animal. European Journal of Social Psychology, 44(3), 187–194. https://doi.org/10.1002/ejsp.2015
Tsarouchi, P., Makris, S., & Chryssolouris, G. (2016). Human–robot interaction review and challenges on task planning and programming. International Journal of Computer Integrated Manufacturing, 29, 916-931. https://doi.org/10.1080/0951192X.2015.1130251
Westerman, D., Edwards, A. P., Edwards, C., Luo, Z., & Spence, P. R. (2020). I-It, I-Thou, I-Robot: The Perceived Humanness of AI in Human-Machine Communication. Communication Studies, 1-16. https://doi.org/10.1080/10510974.2020.1749683
Xu, K., & Lombard, M. (2017). Persuasive computing: Feeling peer pressure from multiple computer agents. Computers in Human Behavior, 74, 152-162.
to frame the potential issues and benefits
n both novel and mundane situations, people form and act on impressions of technology that are based on a longstanding psychological tendency towards anthropomorphizing the physical world
relationships composed of both human and technological partners (e.g., a team with three humans and a machine)
modality, agency, interactivity, and navagability cues affect how people perceive credibility of information
Perceived capabilities of a technology (e.g., Twitter let's you connect with people, Canvas is useful for managing schoolwork)
to the text, audio, video, or other sensory form of communication available in a given technology
who or what is responsible for the message
interaction and activity—and focuses on a given technology’s ability to involve or engage the users
interface features that suggest transportation from one location to another
attentional shortcuts which guide evaluations
treating others differently because of their group memberships
software which helps structure group decision and discussion processes